/TRAIN TO EARN/
Data Collection, Contribution, and Creation in the Train-to-Earn Economy
1. Internet Of Things (IoT) Devices and Granular Data Collection
DePIN networks incentivize users to install, monitor, and operate IoT devices in order to collect data from their physical environment. This data can be used to form large-scale data sets comparable to those collected by central authorities using far fewer resources. Data collected from IoT devices is granular and allows for precise monitoring, analysis, and decision-making based on specific and real-time information. This data, when applied to LLMs either through RHLF fine-tuning, RAG optimization, or Long context models, will allow for autonomous AI responses to real-time data-enabling a live autonomous service layer that is responsive to real-world events.
Decentralized large-scale data collection will outperform centralized methods of granular data collection both in cost efficiency and scale. For example, Google Street View imagery is “outdated by ten years,” whereas Hivemapper (decentralized IoT mapping through dashboard vehicle cameras) contributors build a “real-time view of our world, mapping places that have not been mapped or where Google Street View needs to be updated.” Hivemapper provides the base-case for real-time IoT data. At scale, this format of incentivized, decentralized data collection will enable the creation of region-specific and global real-time datasets. Moreover, train-to-earn incentivized data collection will kick-start research efforts in regions with limited technological infrastructure that currently lack substantial data sets.
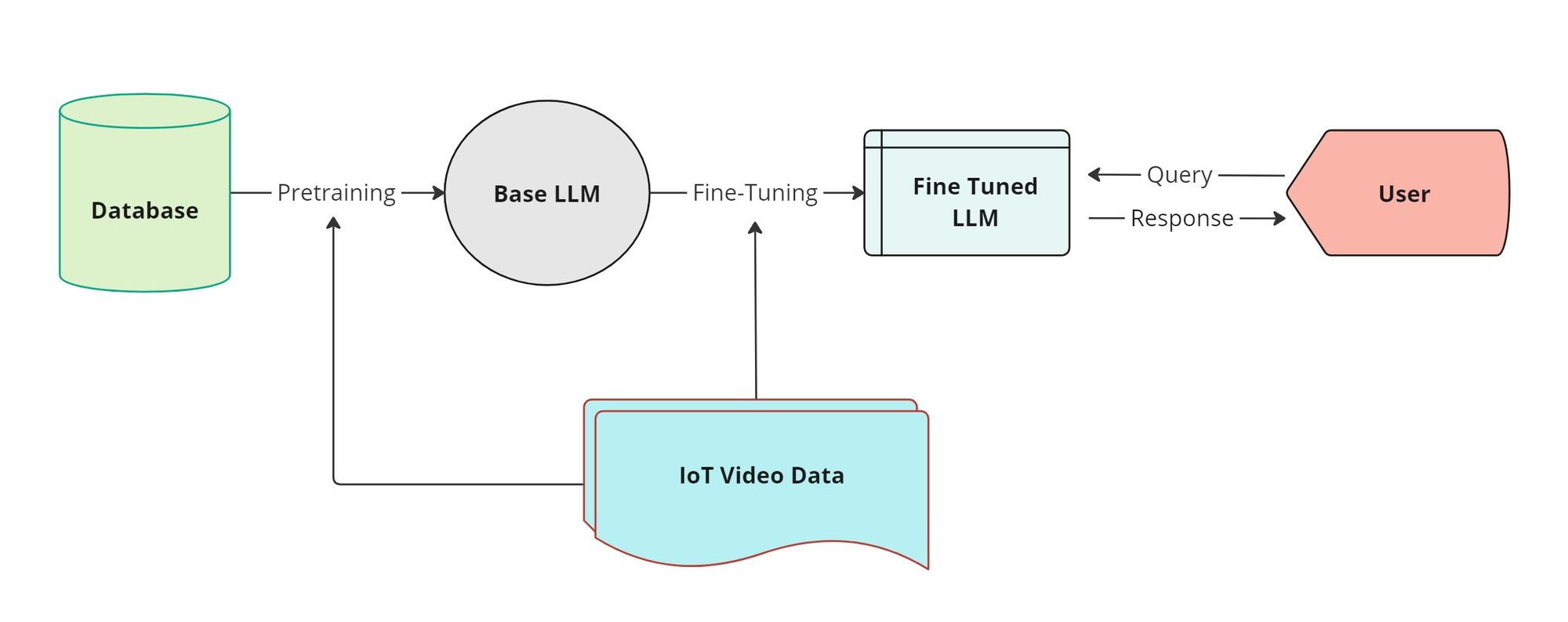
2. Interactive Forms of IoT Data Collection and Contribution
Expanding on automatic methods of IoT data collection, Social-AI applications with incentive structures offer a new paradigm of coordinated and collective IoT data contribution. Personal IoT (smart technologies) device users can contribute permissioned, personal and real-time data to train highly personalized LLM assistants, avatars, and companions. Moreover, this data may be used to generate real-time intelligence for direct training of larger models, or as live accessible data for AI autonomous services.
Coordinated, collective social IoT data will be incentivized through gamified social quests interwoven with the real world. These social quests will be interactive and implicit. They will be couple real-life daily tasks with IoT data collection, monitoring human feedback. They will even capture data from daily labor tasks, combining the world’s workforce labor with IoT data contribution for training purposes. Incentivized individual and coordinated collective forms IoT data contributions will serve as the basis for generating specific natural human feedback data. One current example is the use of Tesla vehicles to help train neural networks for autonomous driving. Here, IoT technology and regular human tasks (driving automobiles) are coupled to generate valuable data for training multi-modal technologies, including AI Agents and Robotic fleets.
3. Decentralized Marketplaces for RHLF Labor
Decentralized data annotation, human feedback, and user testing will also serve as valuable contributions towards pre-training, fine-tuning, and model optimization.
RHLF is considered a "significant advancement in the field of Natural Language Processing (NLP)” Abideen. However, scaling RHLF by gathering human reference data is costly as it involves large amounts of human labor. Decentralized networks that incentivize participants through train-to-earn models may provide a means to economically scale RHLF and decentralize aspects of model pre-training that require routine human labor, human judgment and feedback.
Decentralized marketplaces for RHLF labor can be used to match model builders using open-source model training networks (TAO, NetMind.AI, Arbius) with data contributors who are willing to participate in manual data aggregation labor, data sharing, surveys (prediction markets), events, user feedback, apps, or other routine data acquisition tasks. Additionally, these marketplaces may be useful in generating, non-expert, aggregated data sets that can be accessed by open-source model training networks and applied to generalized models through RAG (KIP Protocol).
4. Data Contribution DAOs: Proprietary Data
Train-to-earn incentives will be used to collect data from specific expert groups, as well as non-expert groups willing to participate in data contribution tasks. Research DAOs may be incentivized to contribute their proprietary (private and expert) data to open-source inference and model training networks either through RAG model optimization or as context for Long context models.
RAG combines generalized models with an “authoritative knowledge based” to optimize model results, whereas Long context models, such as in Google’s Gemini 1.5, accept any data format, code, text, audio and video. In both cases, proprietary data from Data Contribution DAOs will optimize models for legal research, healthcare, financial analysis, cross-lingual applications, data extraction, historical data analysis, and much more.
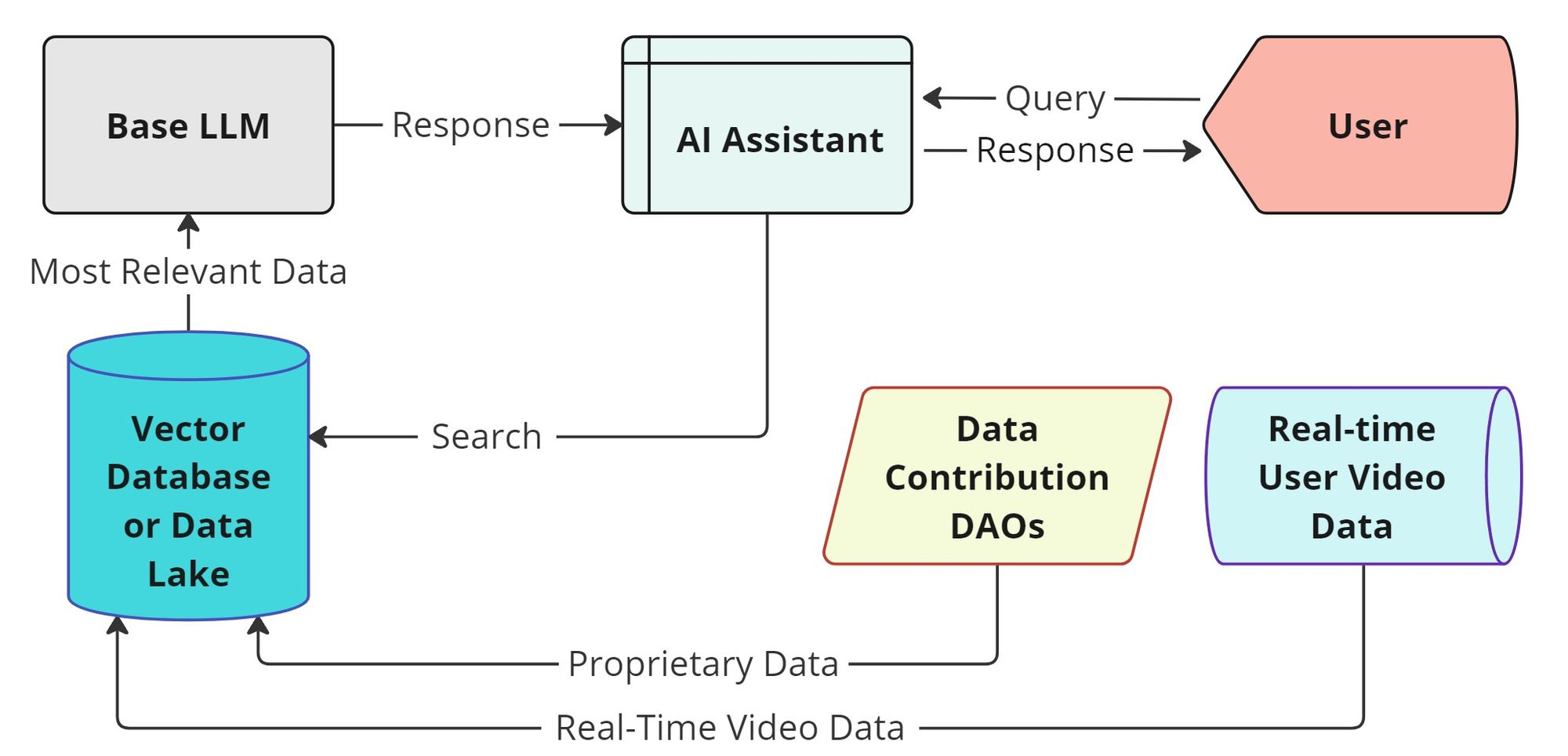
The Multi-Modal Future
As the oil industry expanded pipeline infrastructure, train-to-earn economic structures will incentivize a new data economy. Decentralized IoT data collection and proprietary private data contributions will increase exponentially in value and form the foundation for a multi-modal world.
The Personal AI is the realization of the "invisible computer,” seamless technology embedded in the user's material and digital sphere through external and wearable IoT devices. This social-technological realization is just the beginning. As we integrate multi-modal applications, we lay the foundation for an automated mechanical labor force, advanced robotics and personal AI Meckas (large personal robots) capable of advancing social, technological, and economic needs.
As the automobile revolutionized personal mobility worldwide, the development of personal AI models and future Meckas will expand human autonomy across the universe.